Support for the Graph- and Heuristic based Topology optimization of crash-loaded structures through Reinforcement Learning
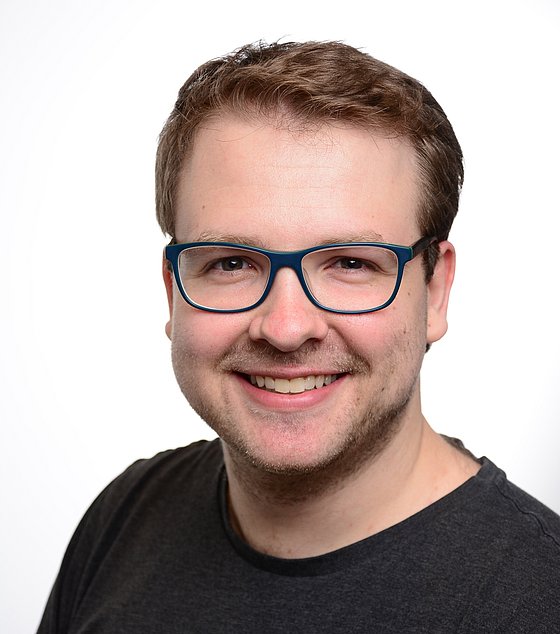
The development of vehicles relies greatly on the design of crash-relevant structural components. However, the optimization of such structures through automated methods is difficult. Purely mathematical optimization methods fail due to the complexity of determining the derivatives of the relevant objective functions and constraints as a function of the design variables. Therefore, the Graph and Heuristic based Topology optimization (GHT) was developed, which guides the optimization process with rules derived from expert knowledge, known as heuristics. The cross-section of extrusion profiles
is described by mathematical graphs within the GHT, allowing the shape and topology of the structure to be easily modified by heuristics. In this dissertation, a novel heuristic is presented that both complements and challenges established expert rules. Using Reinforcement Learning (RL) techniques, the Machine Learning (ML) model underlying the heuristic, called the agent, autonomously learns a strategy to stiffen the structure to be optimized through topological modifications. To ensure a consistent description of local domains within the structure graphs, a cellbased RL environment is introduced. The cell delineates the region within which the heuristic can apply topological modifications. A newly developed shape deviation measure describes the stiffness of a cell geometrically. The agents are trained via a three-stage process, wherein the initial two stages entail a methodical search for appropriate training parameters. Subsequently, in the third stage, the agent’s strategy is further generalized through transfer learning. Beside the stiffening heuristic, the possibility of developing
another RL heuristic based on the presented approach, which makes the structures more compliant, is discussed. The structure stiffening heuristic is investigated in various practical GHT optimizations for
its performance and added value to the GHT. Analyses are carried out on a frame model, a bending beam and a rocker cutout in various crash load cases. In many optimizations it is shown that the RL heuristic can be successfully applied and leads the optimization process to a better structure compared to a benchmark optimization without the RL heuristic.